A new tool for detecting depression stigma on social media has been established by researchers from the Institute of Psychology, Chinese Academy of Sciences (IPCAS), paving the way for more effective stigma reduction campaigns.
According to the World Health Organization, in 2015, the total number of people with depression was estimated to exceed 300 million, equivalent to 4.4% of the global population. Stigma around depression is a major barrier to help seeking behaviors and delays the professional treatment of depression. Therefore, lowering depression stigma is necessary.
Information distributed through social media is an important contributor to the dissemination of material that may increase depression stigma. If the characteristics of such stigma information can be understood, it may be possible to design interventions that counter its negative influence. However, the sheer volume of social media data makes it difficult to identify stigma information by human coders.
In response, Dr. Tingshao Zhu’s research team (Computational CyberPsychology Lab, CCPL) has used big data methods to measure depression stigma automatically on social media (Sina Weibo, a famous social media service in China). The CCPL team downloaded and analyzed a sample of 15,879 pieces of publicly available Weibo posts. Then, using psycho-linguistic analysis and data mining methods, researchers built and tested classification models for detecting psycho-linguistic patterns of depression-related stigmatizing language use in Weibo posts.
Published in the latest issue of Journal of Affective Disorders, the study shows that 6.09% of downloaded Weibo posts reflected depression stigma. The accuracy for differentiating between stigmatizing and non-stigmatizing Weibo posts reached 75.2% (F-Measure value). And the accuracy for differentiating among three specific types of stigmatizing Weibo posts reached 86.2% (F-measure value) (Table 1).
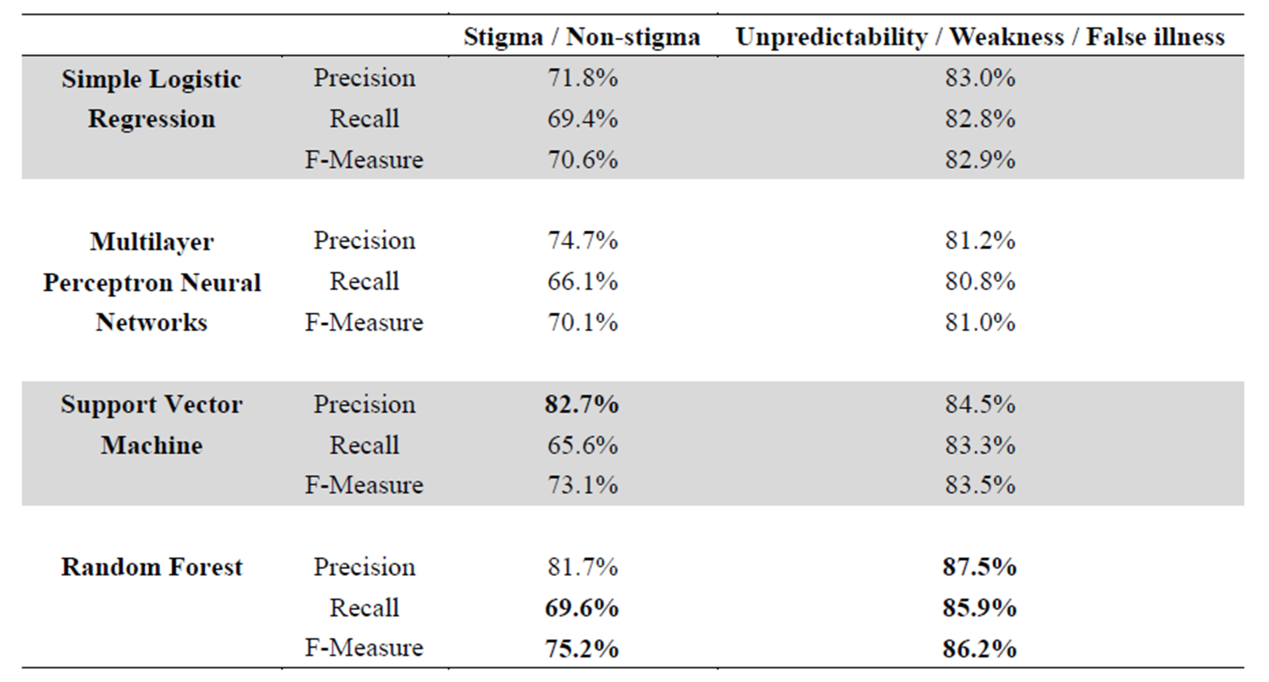
Table 1 Performance of classification models
The results could help improve mental health outcomes by realizing a real-time monitor of stigma information among massive information. “With the help of big data methods, we can detect and track depression stigma information more efficiently. This can help us understand what education is needed for those with stigmatizing attitudes, and know how to design more effective stigma reduction campaigns.” said Dr. Tingshao Zhu.
This work was supported by the National Basic Research Program of China (grant number 2014CB744600).
The paper is now available online at http://www.jad-journal.com/article/S0165-0327(17)32184-5/abstract
Contact:
LI Ang
Beijing Forestry University
angli@bjfu.edu.cn