Magnetic resonance imaging (MRI) is widely used in neuroradiology to detect brain lesions including stroke, vascular disease, and tumor tissue. Still, MRI has been less useful in definitively identifying degenerative diseases including Alzheimer’s disease (AD), mainly because signatures of the disease are diffuse within the images and hard to distinguish from normal aging. Machine learning and deep learning methods have been trained on relatively small datasets, but limited training data often leads to poor generalization performance on new datasets that have not been used in the algorithms’ training.
Constructing deep learning models with good generalizability requires a large amount of training data. Prof. YAN Chao-Gan and his colleagues from Institute of Psychology, Chinese Academy of Sciences, explored a practical brain imaging-based AD classifier with high generalization capability via learning/transfer learning on a diverse range of large-scale datasets. In sum, 85,721 MRI scans of 50,876 participants from more than 217 sites/scanners were applied from public datasets and preprocessed using a standardized pipeline, of which consisted one of the largest sample sizes in brain imaging research.
Based on this unprecedentedly diverse brain imaging sample, a sex classifier served as a base-model for transfer learning to promote model generalizability was trained and achieved about 95% accuracy. After transfer learning, the model fine-tuned to AD achieved 90.9% accuracy in stringent leave-sites-out cross-validation in Alzheimer’s Disease Neuroimaging Initiative (ADNI) and 94.2%/93.6%/91.1% accuracy for direct tests on three unseen independent datasets. Using this classifier to test structural images from patients with mild cognitive impairment (MCI), MCI patients who progressed to AD were more than three times more likely to be predicted to have AD than those who did not (65.2% vs. 20.6%). The relevant sex and AD classifiers have been deployed to an online test site where interested researchers can simply upload raw data or pre-processed gray matter volume and gray matter density maps to obtain the corresponding brain sex or AD discriminant scores (the discriminant scores can only be used for research purposes but not as clinical cues or diagnoses at any circumstance).
In the future, Prof. YAN’s group will further improve the ability of the classifier to predict disease progression. Considering the non-invasive and radiation-free nature of MRI, the MRI-based classifier is expected to supplement or replace invasive and radiation-intensive positron emission tomography (PET) examinations to improve the efficiency of early diagnosis of AD and create greater social value.
The study entitled “A practical Alzheimer’s disease classifier via brain imaging-based deep learning on 85,721 samples” has been published since Oct 13, 2022 in Journal of Big Data.
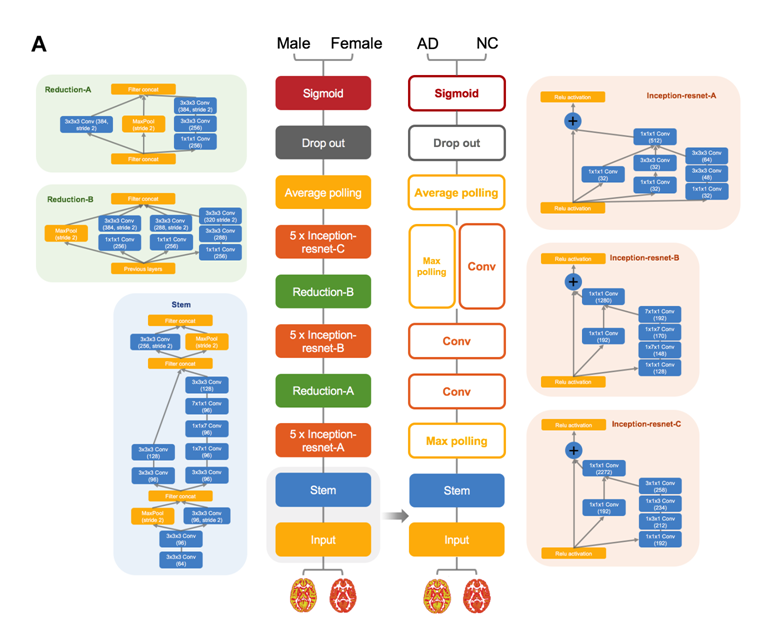
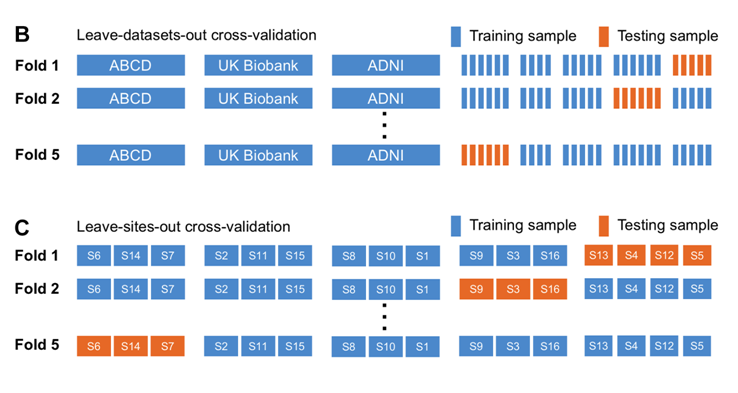
Fig.1 Flow diagram for the Alzheimer’s disease (AD) transfer learning framework and cross-validation procedure. Image by Dr. LU Bin.
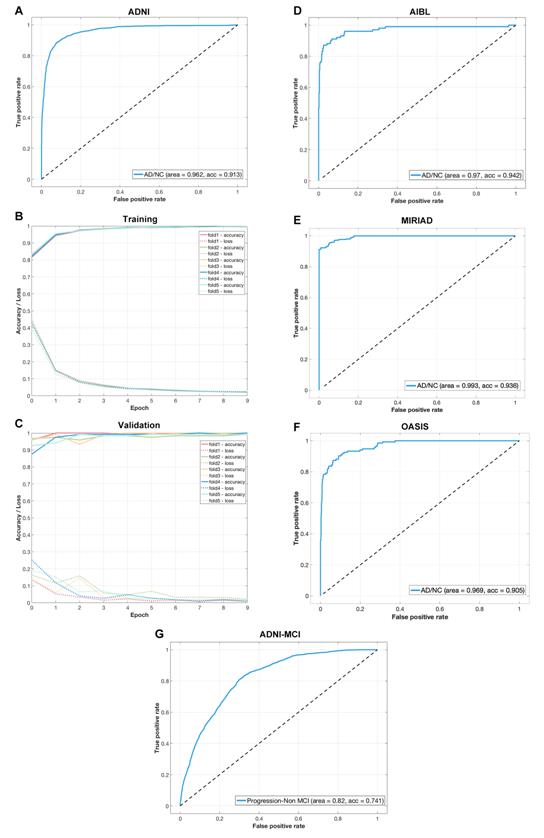
Fig. 2 Performance of the Alzheimer’s disease (AD) classifier. Left panel shows the training and testing performance of the AD classifier on ADNI sample. Right panel shows the testing performance of the AD classifier on independent samples. Image by Dr. LU Bin.
LIU Chen
Institute of Psychology
Chinese Academy of Sciences
Beijing 100101, China.
E-mail: liuc@psych.ac.cn